When One Minute Can Cost You a Million: Predicting Share Prices in Real-Time with Apache Spark and Apache Ignite
The stock trading world is a harsh reality for many investors, in which many times you need to make critical decisions in a very short window of time. In this constantly changing landscape in which prices are constantly updated and investing at the right moment makes all the difference, having the right tools to collect, process and analyze big volumes of data in a short amount of time becomes very important.
This presentation aims at presenting an architecture for a distributed application with in-memory capabilities, in order to collect, process, classify and visualize different equities based on their current and historic prices, aiding stock traders on their investment decisions in real-time.
Due to its technical nature, attendees should have some background in data engineering and architecting, or experience as investors in the stock market.
You will first learn how to set up a set of Kafka producers and brokers, either by using Docker containers or separate instances, to consume price data from multiple equities via one or more APIs, on a minute to minute basis, in a fault tolerant fashion.
The processing phase will come next, in which we will look at the Spark Streaming module as a way to perform parallel in-memory computations on the ingested data and thus optimizing performance, preparing data for storage on an Apache Ignite grid comprised of one or more cached tables, via the Ignite-Spark module, thus providing sub second, SQL like syntax querying access to equity prices.
You will see how all your tables can be persisted to HDFS, followed by a cache cleanup to manage memory usage. At the same time, every five minutes, another Spark application shall perform a classification using its Machine Learning module, for all equities based on current and historic prices, to determine if investors should invest or not.
End users are then finally able to visualize these conclusions with minute to minute updating Tableau charts connected directly to Apache Ignite, which you will learn how to set up as well.
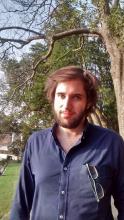
Currently a member of Nomad Tech, leading provider of IoT solutions for the railway industry, developing a Big Data solution for ingestion, processing and storage of train fleets data in near real-time.
Have previously published a paper for ECML-PKDD 2016 in association with Bosch Portugal, for the implementation of a scalable online failure prevention architecture for heating systems.